AI for Health
![[Translate to English:] Medical setup for cancer](https://www.ai-academy.uni-hannover.de/fileadmin/_processed_/5/7/csm_Medical-2_30fca8ec34.jpg)
![[Translate to English:] Medical setup for cancer](https://www.ai-academy.uni-hannover.de/fileadmin/_processed_/5/7/csm_Medical-2_861f81f02a.jpg)
![[Translate to English:] Medical setup for cancer](https://www.ai-academy.uni-hannover.de/fileadmin/_processed_/5/7/csm_Medical-2_725a9b825f.jpg)
Responsible: Prof. Dr. Bodo Rosenhahn
Target group: Students of computer science and electrical and information engineering
Description: The goal is to provide knowledge of the technical fundamentals of current image acquisition systems, elementary image processing and visualisation techniques, as well as essential hardware computing platforms for imaging and image analysis systems. The syllabus includes introduction and motivation, optical image acquisition systems (optics, cameras, formal image definitions), imaging techniques (X-ray, ultrasound, MR, CT, electro-impedance tomography, terahertz imaging), fundamentals of image processing (local and global operators, contrast enhancement, noise and artefact reduction, etc.), fundamentals of visualisation, image segmentation, compression of medical image data, architectures for imaging and image analysis systems, and data formats in medical imaging.
Credits: 5
Language: German
AI for Production Engineering
![[Translate to English:] Production factory](https://www.ai-academy.uni-hannover.de/fileadmin/_processed_/0/2/csm_Production-2_426e9a48ae.jpg)
![[Translate to English:] Production factory](https://www.ai-academy.uni-hannover.de/fileadmin/_processed_/0/2/csm_Production-2_984ab58ec4.jpg)
![[Translate to English:] Production factory](https://www.ai-academy.uni-hannover.de/fileadmin/_processed_/0/2/csm_Production-2_246fd69988.jpg)
Responsible: Prof. Dr. Berend Denkena
Target group: Students of mechanical engineering, mechatronics, industrial engineering, production and logistics, and computer science.
Description: The course gives participants an insight into the application of artificial intelligence (AI) methods in production. Building on an introduction to production engineering processes and issues, participants learn about areas of application of AI using practical examples (e.g. wear detection, anomaly detection for quality assurance, and adaptive process planning). The theoretical units are offered as block courses and are supplemented by practical exercises in which the participants design their own solution approaches in interdisciplinary groups.
Credits: 5
Language: German
Spatial data science

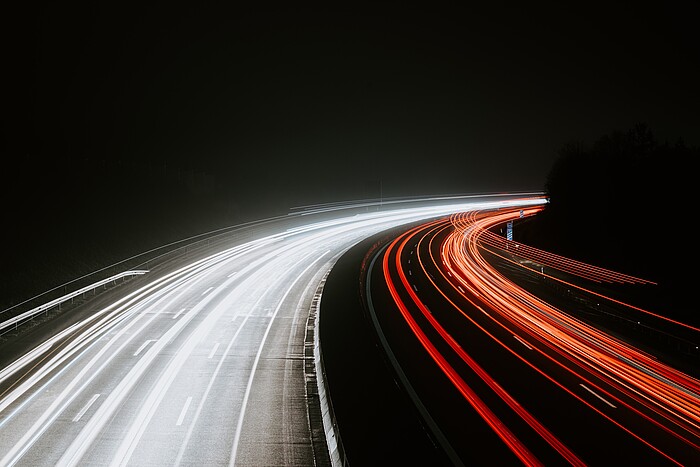

Responsible: Prof. Dr. Monika Sester
Target group: All
Description: Students acquire basic concepts and methods for analyzing spatial and spatio-temporal data. In thematically organized sections, they gain in-depth insights into central aspects of the subject area. The topic “Spatial Query Languages” deals with the introduction of spatial operators and topological relations, which play an important role in the analysis of spatial data sets. In the topic “Multiple Representation”, the representation of spatial data on different scales is explained and various approaches to generalization are discussed. Another focus is on the use of artificial intelligence methods, in particular machine learning methods, to recognize spatial patterns and perform complex analysis tasks. In addition, the topic of “spatio-temporal analyses” is dedicated to the integration of the temporal dimension, for example to identify time-dependent patterns in motion data.
The accompanying practical exercise enables students to put the theoretical content into practice. They implement algorithms independently and apply them to real data sets. This practice-oriented approach promotes an in-depth understanding of the potential and challenges of the methods learned.
Credits: 5
Language: English
Image Analysis I
![[Translate to English:] A picture of a houses organized](https://www.ai-academy.uni-hannover.de/fileadmin/_processed_/5/c/csm_Geo-_15b993871c.jpg)
![[Translate to English:] A picture of a houses organized](https://www.ai-academy.uni-hannover.de/fileadmin/_processed_/5/c/csm_Geo-_12ab7f460d.jpg)
![[Translate to English:] A picture of a houses organized](https://www.ai-academy.uni-hannover.de/fileadmin/_processed_/5/c/csm_Geo-_55d26e8516.jpg)
Responsible: Prof. Dr. Franz Rottensteiner
Target group: Students of geodesy and geoinformatics
Description: Students learn modern supervised methods of classifying image and surface data with a focus on strategies for their use for the acquisition and updating of geodata and other applications from geoinformatics. Among other ML methods, Deep Learning methods, especially Convolutional Neural Networks (CNN) are discussed and applied. A particular focus is on strategies to reduce the need for hand-labeled training data, especially methods for domain adaptation and learning with erroneous training data. The syllabus includes Introduction: sensors and data acquisition in remote sensing, georeferencing of remote sensing data, hand-crafted features; Methods for Machine Learning and Deep Learning for image analysis; Deep domain adaptation and learning under label noise; Topographic applications of CNN: classification of land cover and land use for acquiring and updating geospatial databases; and Other applications of CNN for image analysis with examples from autonomous driving and the preservation of cultural heritage.
Credits: 5
Language: English
AI in Teacher Education
![[Translate to English:] the word 'TEACH' written using scrabble blocks](https://www.ai-academy.uni-hannover.de/fileadmin/_processed_/a/a/csm_AI-Education_22d7ca62e0.jpg)
![[Translate to English:] the word 'TEACH' written using scrabble blocks](https://www.ai-academy.uni-hannover.de/fileadmin/_processed_/a/a/csm_AI-Education_40e0f88ce9.jpg)
![[Translate to English:] the word 'TEACH' written using scrabble blocks](https://www.ai-academy.uni-hannover.de/fileadmin/_processed_/a/a/csm_AI-Education_dee14abc0b.jpg)
Responsible: Prof. Dr. Sascha Schanze
Target group: Students of teacher training programs
Description: Innovative forms of AI-supported learning support enable new assessment and diagnostic pathways. Learner data are to be viewed as footprints of a learning journey and take on an ambiguous character in analysis and interpretation. A core goal of the module is to professionalise prospective teachers to understand procedures of data-based learning and to integrate them appropriately into their own teaching practice. Likewise, impulses for content in corresponding specialisation modules are to be given to develop applications for the educational context.
Credits: Introductory course 2CP, thereafter through fundamentals and specialisation 5 CP
Language: German
AI for Education



Responsible: Prof. Dr. Steffi Robak
Target group: Students of the Master of Education; Engineering Science courses
Description: The module provides an introduction to the central application fields of artificial intelligence for the design of innovative educational processes in continuing education and the treatment of both technological and pedagogical issues associated with the use of technologies. In the interdisciplinary consideration of the application field, the way in which technologies are used refers to implicit learning-theory-oriented concepts of education and learning. Control and monitoring fantasies of learning processes get a renaissance, which has to be critically considered under the demanded "primacy of pedagogy", especially since the increasing orientation towards competencies and the demand for autonomy/self-control of learning processes is rather based on constructivist assumptions. The module thus builds competencies for the development and use of trustworthy AI for the design of lifelong learning processes for both future continuing education staff and students of technical sciences. The syllabus includes but is not limited to, Intelligent Search Systems, Personalized Recommender Systems, Intelligent Tutoring Agents and Assistance Systems, Voice Assistants/Chatbots, Automated Performance Assessment/Balancing, and Learning Theories.
Credits: 5
Language: German
Data Science for Design and Engineering



Responsible: Prof. Philipp Geyer, Mr. Shaofan Wang
Target group: master students from the Leibniz AI Academy, all engineering disciplines, landscape architecture and environmental planning, as well as computer science
Description: Due to the need for sustainable design and the resulting complexity in diverse fields, professionals from various disciplines face the critical challenge of making effective design decisions, e.g., in building engineering, automotive engineering, mechanical engineering, civil engineering, etc. This course tackles this challenge by building skills in the application of data science and artificial intelligence in decision-making contexts. Based on paradigms of systems engineering and design space exploration (DSE), the course guides the participants to apply cutting-edge data science and AI techniques, such as machine learning and clustering, in such contexts. These techniques will be harnessed in design projects to integrate the necessary examination and understanding of variants (DSE) and the required engineering for sustainability in decision-making. Ultimately, it positions AI as a powerful tool assisting designers and engineers with comprehensive strategic information in the quest for sustainable and efficient solutions.
Credits: 5
Language: English